Single-Chip Cloud Computer (SCC) from Intel
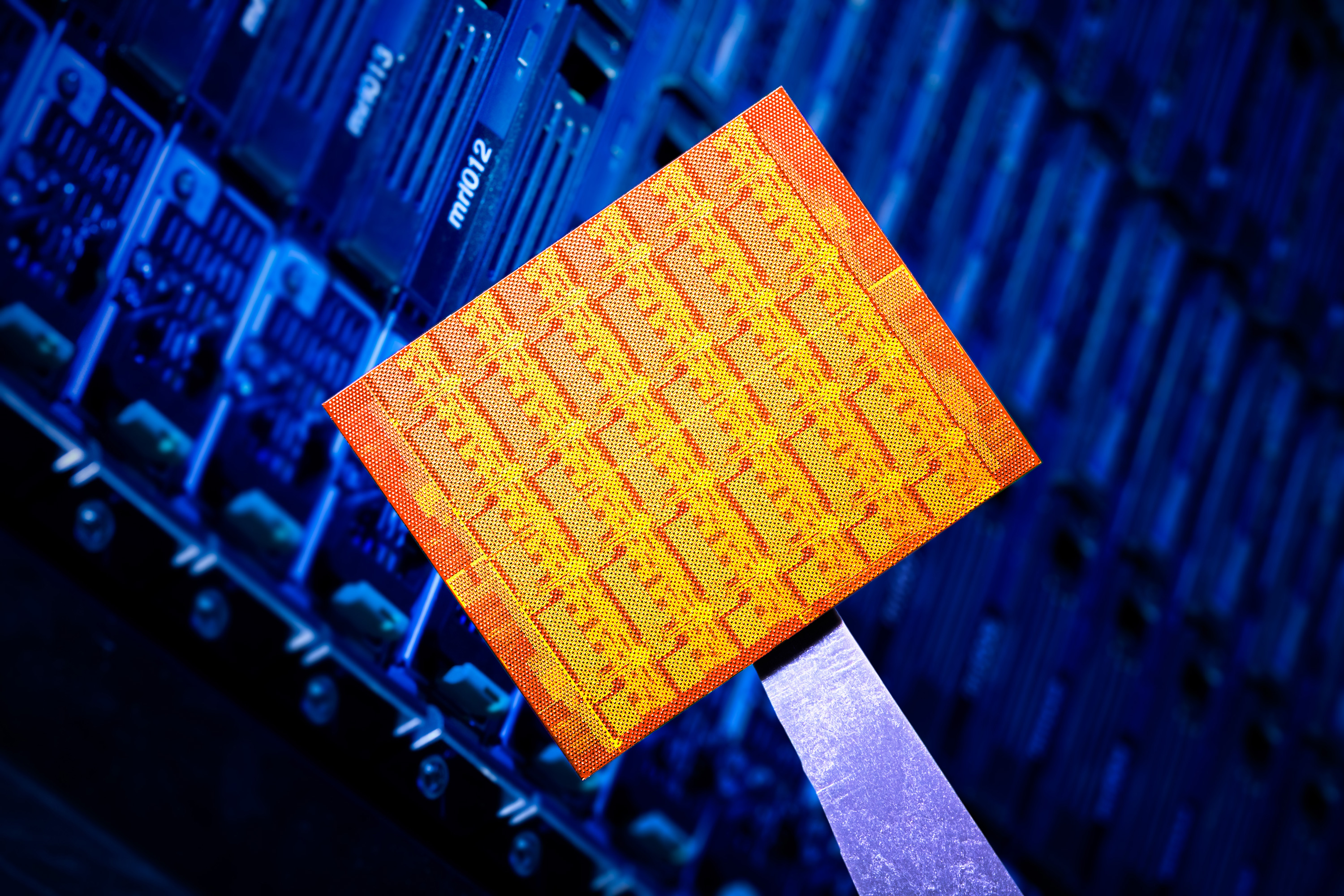
In the project we want to study how to perform intelligent resource management in cloud-computing environment, in this case, on Intel Single Chip Cloud Computer (SCC) platform. Different programs/applications have different resource need in different phase of execution. We want to explore the possibility of dynamic resource management and evaluate the system throughput on SCC. The research hypothesis is that based on the need of a certain job during a certain phase, we should be able adjust the number of resources in the cloud that dedicated to that job. We try to verify it through implementing the intelligent resource management on SCC. If the hypothesis is verified, it would have broad impact on how to utilize the future cloud computing platform to achieve the best system resource utilization and throughput. We expect to be able to generate explicit data regarding the performance of the intelligent resource management of our scheme on SCC, comparing with the case without intelligent control. The results would benefits Intel and the industry in how to maximize the performance and system throughput of future many-core architecture and cloud-computing platform; and the knowledge generated will benefit the greater research community as a whole.
Related Publications
SmartWorld 2018
Energy-Aware Automatic Tuning of Many-Core Platform via Gradient Descent (ieee)
Samer Akiki, Zhiliu Yang, Chen Liu, Jie Tang, Shaoshan Liu.
2018 IEEE SmartWorld, Ubiquitous Intelligence & Computing, Advanced & Trusted Computing, Scalable Computing & Communications, Cloud & Big Data Computing, Internet of People and Smart City Innovation (SmartWorld/SCALCOM/ UIC/ATC/CBDCom/IOP/SCI), Guangzhou, China, October 8-12, 2018, pp.258-265
Abstract: Even though attaining high performance has been the user's pursuit traditionally, in the many-core era, the emphasis has shifted towards controlling the power and energy consumption, so as to maintain a satisfying performance while consuming an acceptable amount of energy. This paper describes an auto-tuning algorithm for the energy efficiency optimization of many-core platform, in this case, a Graphic Processing Unit (GPU). We employed gradient descent algorithm as the basis for this optimization. Metrics such as energy and energy delay product (EDP) are examined using programs representing different types of workloads such as sequential, parallel and hybrid. Based on the experimental results, our method achieves the level of savings over 15% in terms of energy consumption when compared with the default on-board governors that also adjust the voltage and frequency of the GPU. Our approach shows an advantage when optimizing towards EDP as well. This shows the effectiveness of our proposed approach.
IPDPSW 2018
GreedyTalents: An Energy-Aware Auto-Tuning Method for Many-Core Processor (ieee)
Tim Platt, Chen Liu, and Zhiliu Yang.
2018 IEEE International Parallel and Distributed Processing Symposium Workshops (IPDPSW 2018)
Vancouver, BC, Canada, May 25, 2018, pp.1076-1083
Abstract: With the era of many-core processing upon us, it is important to be able to effectively utilize the abundant number of cores available in the processor. Given multiple programs to be run simultaneously, one must decide not only how many cores to allocate to each program, but also at what frequency and voltage to run them. Furthermore, the goal of producing the best performance and the lowest energy consumption or the lowest energy delay product (EDP), without any knowledge about the programs, this can be a challenging task. For programs that can take advantage of multiple cores, what we observed is that the largest benefit in reducing the execution time and EDP is by allowing the program to use additional cores. This execution time advantage can follow an exponentially decaying function, thus providing an effective mechanism in determining the number of cores to use for each program. In this paper we introduce a heuristic method - GreedyTalents - to accomplish this task based on the empirical observation of the execution time of the running programs. We also show that GreedyTalents provides equal or better results than other comparable methods, and converges faster in determining the settings to use, showing it as a promising method of energy-aware auto-tuning for many-core processors.
SCPE
Automatic Tuning on Many-Core Platform for Energy Efficiency via Support Vector Machine Enhanced Differential Evolution (SCPE)
Zhiliu Yang, Zachary I. Rauen, Chen Liu
Scalable Computing: Practice and Experience, Volume 18, issue 2, pp.117-131, 2017
DOI: 10.12694/scpe.v18i2.1284
Abstract: The modern era of computing involves increasing the core count of the processor, which in turn increases the energy usage of the processor. How to identify the most energy-efficient way of running a multiple-program workload on a many-core processor while still maintaining a satisfactory performance level is always a challenge. Automatic tuning on the voltage and frequency level of a many-core processor is an effective method to aid solving this dilemma. The metrics we focus on optimizing are energy usage and energy-delay product (EDP). To this end, we propose SVM-JADE, a machine learning enhanced version of an adaptive differential evolution algorithm (JADE). We monitor the energy and EDP values of different voltage and frequency combinations of the cores, or power islands, as the algorithm evolves through generations. By adding a well-tuned support vector machine (SVM) to JADE, creating SVM-JADE, we are able to achieve energy-aware computing on many-core platform when running multiple-program workloads. Our experimental results show that our algorithm can further improve the energy by 8.3% and further improve EDP by 7.7% than JADE. Besides, in both EDP-based and energy-based fitness SVM-JADE converges faster than JADE. Parallel tree skeletons are basic computational patterns that can be used to develop parallel programs for manipulating trees. In this paper, we propose an efficient implementation of parallel tree skeletons on distributed-memory parallel computers. In our implementation, we divide a binary tree to segments based on the idea of m-bridges with high locality, and represent local segments as serialized arrays for high sequential performance. We furthermore develop a cost model for our implementation of parallel tree skeletons. We confirm the efficacy of our implementation with several experiments.
IJES
EEG Processing: A Many-Core Approach Utilizing the Intel Single-Chip Cloud Computer Platform (Inderscience)
Gildo Torres, Paul McCall, Chen Liu, Mercedes Cabrerizo, and Malek Adjouadi
International Journal of Embedded Systems (IJES), Volume 9, Issue 5, pp.464-474, 2017
DOI: 10.1504/IJES.2017.086720
Abstract: Epilepsy is the most frequent neurological disorder other than stroke. The electroencephalogram (EEG) is the main tool used in monitoring and recording brain signals. In this study, we target two detection algorithms that are essential in the diagnosis of epileptic patients. These algorithms detect high frequency oscillations (HFO) and interictal spikes (IIS) in subdural EEG recordings respectively. This paper presents the efforts on porting both EEG processing algorithms into Intel's concept vehicle, the single-chip cloud computer (SCC), a fully programmable 48-core prototype provided with an on-chip network along with advanced power management technologies and support for message-passing. Several experiments are presented for different SCC configurations, where we vary the number of cores used and their respective voltage/frequency settings. The application was decomposed into two execution regions (i.e., load and execution). Results are presented in the form of performance, power, energy, and energy-delay product (EDP) metrics for each experiment.
NAPS 2015
Parallel Gaussian Elimination on Single-Chip Cloud Computer (ieee)
Yamin Wang, Chenxi Dai, Chen Liu, and Lei Wu
North America Power Symposium (NAPS) 2015, UNC Charlotte, October 4-6, 2015
DOI: 10.1109/NAPS.2015.7335094
Abstract: Gaussian elimination (GE) method is widely used in the solution of systems of linear equations. The Single-chip Cloud Computer (SCC) is an experimental processor created by Intel Labs. This paper analyzes performance of parallel Gaussian Elimination method applied on SCC. Three systems of equations with different sizes are tested. The results show that Gaussian elimination method can benefit from the multi-core systems and better performance can be achieved when the size of systems becomes larger.
M2A2 2015
Parallel BP Neural Network on Single-chip Cloud Computer (ieee)
Boyang Li and Chen Liu
The 7th IEEE International Workshop on Multicore and Multithreaded Architectures and Algorithms (M2A2 2015), in conjunction with HPCC 2015, August 24-26, 2015, New York, USA
DOI: 10.1109/HPCC-CSS-ICESS.2015.280
Abstract: Neural network has been a clear focus in machine learning area. Back propagation (BP) method is frequently used in neural network training. In this work we paralleled BP neural network on Single-Chip Cloud Computer (SCC), an experimental processor created by Intel Labs, and analyzed multiple metrics under different configurations. We also varied the number of neurons (nodes) in the hidden layer of the BP neural networks and studied the impact. The experiment results show that a better performance can be obtained with SCC, especially when there are more nodes in the hidden layer of BP neural network. A low voltage and frequency configuration contributes to a low power per speedup. What is more, a medium voltage and frequency configuration contributes to both a low energy consumption and energy-delay product.
JPDC
How Many Cores do We Need to Run a Parallel Workload? A Test Drive of the Intel SCC Platform (ScienceDirect))
Chen Liu, Pollawat Thanarungroj, and Jean-Luc Gaudiot
Journal of Parallel and Distributed Computing (JPDC) , Vol. 74, Issue 7, Pages 2582-2595, July 2014
DOI:10.1016/j.jpdc.2013.12.011
Abstract: As semiconductor manufacturing technology continues to improve, it is possible to integrate more and more transistors onto a single processor. Many-core processor design has resulted in part from the search to utilize this enormous transistor real estate. The Single-Chip Cloud Computer (SCC) is an experimental many-core processor created by Intel Labs. In this paper we present a study in which we analyze this innovative many-core system by running several workloads with distinctive parallelism characteristics. We investigate the effect on system performance by monitoring specific hardware performance counters. Then, we experiment on varying different hardware configuration parameters such as number of cores, clock frequency and voltage levels. We execute the chosen workloads and collect the timing, power consumption and energy consumption information on such a many-core research platform. Thus, we can comprehensively analyze the behavior and scalability of the Intel SCC system with the introduced workload in terms of performance and energy consumption. Our results show that the profiled parallel workload execution has a communication bottleneck on the Intel SCC system. Moreover, our results indicate that we should carefully choose the number of cores to execute different workloads in order to yield a balance between execution performance and energy efficiency for different applications.
JSPS
An Auto-tuning Assisted Power-Aware Study of Iris Matching Algorithm on Intel's SCC (springer)
Gildo Torres, Chen Liu, Jed Kao-Tung Chang, Fang Hua, and Stephanie Schuckers
The Journal of Signal Processing Systems (JSPS), May 2014
DOI: 10.1007/s11265-014-0901-4
Abstract: Biometric applications become paramount across private sectors, industry, as well as government agencies. As large amount of data being collected from many different sources, managing such volumes of data and developing efficient and effective large-scale operational solutions have become a concern. For example, real-time identification of individuals with the purpose of allowing or denying them access to specific systems or resource is challenging from the performance point of view. In addition, processing large amounts of data requires a significant amount of energy. The Single-chip Cloud Computer (SCC) is an experimental processor created by Intel Labs. In this paper we employ SCC, which supports different configurations in terms of number of cores, frequency, and voltage settings, to investigate the power-aware computing and performance enhancement of an iris matching algorithm on such many-core architecture. This biometric application contains a large degree of parallelism that can be exploited by porting it onto the SCC. Various metrics such as performance, power, energy, energy delay product (EDP), and power per speedup (PPS) are studied when executing the application under different SCC configurations. We also analyze how the results of these metrics vary as we change different parameters. In the latest stage of this study, we apply an auto-tuning approach based on Differential Evolution (DE) algorithm in an effort to quickly approaching the optimal configuration of the SCC based on the targeted metric. This allows us to traverse only a portion of the search space. Such approach proves to be very useful for energy-related metrics.
SPLASHMARC 2013
Two-Dimensional Convolution on the SCC (ResearcGate)
David Illig and Chen Liu
2013 Many-Core Architecture Research Community Symposium (SPLASHMARC 2013), in conjunction with SPLASH 2013, Indianapolis, Indiana, USA, October 28, 2013
Abstract: Convolution is one of the most widely used digital signal processing operations. This work aims to distribute two-dimensional convolution operation across Intel’s Single-Chip Cloud Computer (SCC), an experimental processor created by Intel Labs. This platform enables experiments with varying both the data sizes and the physical parameters of the platform such as voltage, frequency, and number of cores. The program can also be optimized subject to power and energy considerations. We find that implementing the convolution operation on the SCC can reduce the calculation time but results in a communication bottleneck. We find that calculations should be run at a lower frequency to reduce energy consumption, while communications should be run at a higher frequency to reduce execution time. Current applications are in the area of early vision using a Gaussian pyramid, while we aim to expand the study to additional image processing areas.
ICPP-EMS 2013
A Power-Aware Study of Iris Matching Algorithms on Intel's SCC (ieee, acm)
Gildo Torres, Jed Kao-Tung Chang, Fang Hua, Chen Liu, and Stephanie Schuckers
The 2013 International Workshop on Embedded Multicore Systems (ICPP-EMS 2013), in conjunction with ICPP 2013, Lyon, France, October 1-4, 2013
DOI: 10.1109/ICPP.2013.122
Abstract: Biometric applications become paramount across private sectors, industry, as well as government agencies. As large amount of data being collected from many different sources, managing such volumes of data and developing efficient and effective large-scale operational solutions are becoming a concern. For example, real-time identification of individuals with the purpose of allowing or denying their access to specific system or resource is challenging from the performance point of view. In addition, processing large amount of data would definitely consume a significant amount of energy. The Single-chip Cloud Computer (SCC) is an experimental processor created by Intel Labs. In this paper we employ SCC, which supports dynamic frequency and voltage scaling (DVFS), to investigate the power-aware computing and performance enhancement of an iris matching algorithm on such many-core architecture. This biometric application contains a large degree of parallelism that we can exploit by porting it onto the SCC. Results in terms of performance, power, energy, energy delay product (EDP), and power per speedup (PPS) metrics of executing the iris matching application under different number of cores, frequency, and voltage settings of the SCC platform are presented. We also analyze how the results for these metrics vary as we change these parameters.
IEEE IGCC 2013
Auto-Tuning Multi-Programmed Workload on the SCC (ieee)
Brian Roscoe, Mathias Herlev, and Chen Liu
The 3rd International Workshop on Power Measurement and Profiling (PMP 2013), in conjunction with IEEE IGCC 2013, Arlington, VA, USA, June 27-29, 2013
DOI: 10.1109/IGCC.2013.6604486
Abstract: The need for power-aware computing has become increasingly apparent. Common power-aware platforms have placed the burden of optimizing energy consumption on the programmer. In many cases this is a complex task which requires more time from the programmer than is acceptable. Hence, auto-tuning for power-aware computing has been proposed to alleviate the programmer from this task. Previous research has been focusing on automatic tuning of individual applications. However, there has been little work that tunes multiple programs across an entire platform. The Single-Chip Cloud Computer (SCC) is an experimental processor created by Intel Labs. In this paper, we present a method that extends auto-tuning to consider the multi-programmed workload across the entire many-core platform of SCC. Using an algorithm based on Differential Evolution, we were able to reduce the energy-delay product of the workload by 58.5%.
ADAPT 2013
Application-Level Voltage and Frequency Tuning of Multi-Phase Program on the SCC (acm)
Kenneth Berry, Felipe Navarro, and Chen Liu
The 3rd International Workshop on Adaptive Self-tuning Computing Systems (ADAPT'13), co-located with HiPEAC 2013, Berlin, Germany, January 22, 2013
DOI: 10.1145/2484904.2484905
Abstract: With the technology advancement, we are quickly progressing towards the many-core era. Corresponding to this shift, techniques on how we program such chip are beginning to change. The Single-Chip Cloud Computer (SCC) is an experimental processor created by Intel Labs. When programmer is given direct control over the frequency and voltage of the cores, ideally we want to identify the phases of the program based on their computation intensity and associate frequency and voltage configuration correspondingly. In order to achieve power and energy saving in this way, however, we need to search through the entire domain of various voltage and frequency combinations supported by the chip, which is a daunting task. In this study, we propose to employ two popular optimization algorithms, i.e., Differential Evolution and Nelder-Mead Simplex, to help identifying the best configuration corresponding to various metrics, i.e., execution time, power, energy, and energy-delay product (EDP). Our experimental evaluation shows that, with a large search space of possible combinations, we can identify the configuration that provides the best result for each specific metric, which aids the tuning for individual phases.
JCST
Pinned OS/Services: A Case Study of XML Parsing on Intel SCC (springer)
Jie Tang, Pollawat Thanarungroj, Chen Liu, Shaoshan Liu, Zhiming Gu, and Jean-Luc Gaudiot
Journal of Computer Science and Technology (JCST), Vol. 28, No. 1, pp.3-13, 2013
DOI: 10.1007/s11390-013-1308-6
Abstract: Nowadays, we are heading towards integrating hundreds to thousands of cores on a single chip. However, traditional system software and middleware are not well suited to manage and provide services at such large scale. To improve the scalability and adaptability of operating system and middleware services on future many-core platform, we propose the pinned OS/services. By porting each OS and runtime system (middleware) service to a separate core (special hardware acceleration), we expect to achieve maximal performance gain and energy efficiency in many-core environments. As a case study, we target on XML (Extensible Markup Language), the commonly used data transfer/store standard in the world. We have successfully implemented and evaluated the design of porting XML parsing service onto Intel 48-core Single-Chip Cloud Computer (SCC) platform. The results show that it can provide considerable energy saving. However, we also identified heavy performance penalties introduced from memory side, making the parsing service bloated. Hence, as a further step, we propose the memory-side hardware accelerator for XML parsing. With specified hardware design, we can further enhance the performance gain and energy efficiency, where the performance can be improved by 20 % with 12.27 % energy reduction.
ARISR 2012
High-Performance Implementation and Evaluation of Blowfish Cryptographic Algorithm on Single-Chip Cloud Computer: A Pipelined Approach (ResearchGate)
Kamak Ebadi, Victor Pena, and Chen Liu
The 2nd International Conference on Applied and Theoretical Information Systems Research (ATISR 2012), Taipei, Taiwan, December 27-29, 2012
Abstract: In the era of modern data communications, as the need for data security arises, the need to reduce the execution time and computation overhead associated with the execution of cryptographic algorithms increases correspondingly. Parallelizing the computation of cryptographic algorithms on many-core computing platforms can be a promising approach to reduce the execution time and eventually the energy consumption of such algorithms. In this paper, we build a pipelined model to analyze and compare the execution time and energy consumption of the Blowfish cryptographic algorithm on the Single-Chip Cloud Computer (SCC), an experimental processor created by Intel Labs. In this model the Blowfish cryptographic algorithm is divided to smaller chunks and each chunk is run only by one core. Using message passing interface, the input data passes in turn through all the cores involved. Due to the communication overhead and latency associated with this model, we experimented and identified the optimal message size to pass between the cores to avoid saturating the on-chip communication network. Our results illustrate that our parallel approach is 27X faster than the sequential approach and yields close to 16X less energy consumption on the SCC platform.
HPPAC 2012
Power-Efficient Schemes Via Workload Characterization on the Intel's Single-chip Cloud Computer (ieee, acm)
Gustavo Chaparro-Baquero, Qi Zhou, Chen Liu, Jie Tang, and Shaoshan Liu
The 8th Workshop on High-Performance, Power-Aware Computing (HPPAC 2012), in conjunction with IPDPS 2012, Shanghai, China, May 21, 2012
DOI: 10.1109/IPDPSW.2012.122
Abstract: The objective of this work is to evaluate the viability of implementing workload-aware dynamic power management schemes on a many-core platform, aiming at reducing power consumption for high performance computing (HPC) application. Two approaches were proposed to achieve the desired target. First approach is an off-line scheduling scheme where core voltage and frequency are set up beforehand based on the workload characterization of the application. The second approach is an on-line scheduling scheme, where core voltage and frequency are controlled based on a workload detection algorithm. Experiments were conducted using the 48-core Intel Single-chip Cloud Computer (SCC), running a parallelized Fire Spread Monte Carlo Simulation program. Both schemes were compared against a performance-driven, but non-power-aware management scheme. The results indicate that our schemes are able to reduce the power consumption up to 29\% with mild impact on the system performance.
IEEE SoutheastCon 2012
A manual approach and analysis of Voltage and Frequency Scaling using SCC (ieee)
Kenneth Berry, Felipe Navarro, and Chen Liu
IEEE SoutheastCon 2012, Orlando, Florida, March 15-18, 2012
DOI: 10.1109/SECon.2012.6197073
Abstract: The current trend of Dynamic Voltage and Frequency Scaling (DVFS) techniques involve algorithms that predict when a processor is in a period of accessing off chip memory and dial down its voltage/frequency during this phase in order to reduce energy consumption with minimal, if any, effect on execution time. These algorithms often operate with a parameter that defines the tolerable performance degradation, because the various operating frequencies that a processor can be set to are often limited. This limit makes it practically impossible to dial down a processor's frequency to the exact optimal frequency that will provide maximal energy efficiency but not affect performance. This leads to a need for these algorithms to include the previously stated parameter to identify cases where choices which degrade performance to an unacceptable level and/or without providing a benefit in energy consumption are avoided. However, the overhead costs incurred by the process of voltage and frequency scaling must also be taken into consideration. We propose a study to determine the impact of these overhead costs on the overall benefit of dynamic voltage and frequency scaling.
IEEE SoutheastCon 2012
Power and Energy Analysis on Intel Single-chip Cloud Computer System (ieee)
Shi Sha, Jiawei Zhou, Chen Liu, and Gang Quan
IEEE SoutheastCon 2012, Orlando, Florida, March 15-18, 2012
DOI: 10.1109/SECon.2012.6197074
Abstract: Improving the computing performance of the multicore and many-core systems is one of the primary interests to computer architecture researchers currently. Message Passing Interface (MPI) and Multi-core techniques are converged to solve this problem. With performance enhancement, the power and energy consumption increase correspondingly. The Single-Chip Cloud Computer (SCC) is an experimental processor created by Intel Labs. This paper proposed an approach to study the power and energy consumption on the 48-core SCC many-core system and realized the message passing on the SCC. First, we profile the execution time, voltage and current on each running set. Later, we calculated the power and energy consumption, and compared them with increasing number of cores, varying voltage and frequency levels. Finally, we reached a conclusion focus on its scalability and relationship between power/energy consumption and system performance in terms of execution time.
IPCCC 2011
Power and Energy Consumption Analysis on Intel SCC Many-Core System (ieee, acm)
Pollawat Thanarungroj and Chen Liu
The 30th International Performance Computing and Communications Conference (IPCCC 2011), Orlando, Florida, November 17-19, 2011
DOI: 10.1109/PCCC.2011.6108095
Abstract: As semiconductor manufacturing technology continues to scale down, it is possible to integrate more transistors into a processor, which gives birth to many-core processor design. This paper introduces an approach to analyze the power and energy consumption of a many-core research platform. The investigation has been done by using the Intel SCC system as an experimental platform. The approach is to collect the time and power profiling of an executing parallel application on the Intel SCC system. And then, the total energy consumed by the entire execution is calculated as a consequence. We studied the effects of power and energy consumption in many-core systems by varying different hardware configuration parameters such as number of cores, clock frequency and voltage level. Thus, the many-core system can be explored for its scalability and fitness in operational cost and performance.